The AI Race Expands: Qualcomm Reveals “Cloud AI 100” Family of Datacenter AI Inference Accelerators for 2020
by Ryan Smith on April 9, 2019 12:30 PM EST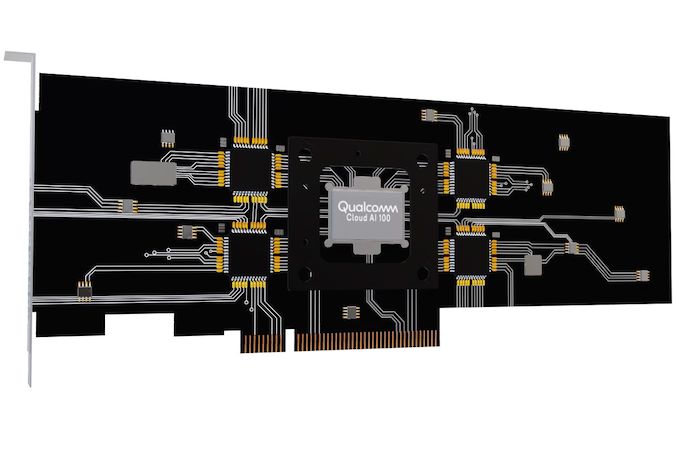
The impact that advances in convolutional neural networking and other artificial intelligence technologies have made to the processor landscape in the last decade is unescapable. AI has become the buzzword, the catalyst, the thing that all processor makers want a piece of, and that all software vendors are eager to invest in to develop new features and new functionality. A market that outright didn’t exist at the start of this decade has over the last few years become a center of research and revenue, and already some processor vendors have built small empires out of it.
But this modern era of AI is still in its early days and the market has yet to find a ceiling; datacenters continue to buy AI accelerators in bulk, and deployment of the tech is increasingly ratcheting up in consumer processors as well. In a market that many believe is still up for grabs, processor markers across the globe are trying to figure out how they can become the dominant force in one of the greatest new processor markets in a generation. In short, the AI gold rush is in full swing, and right now everyone is lining up to sell the pickaxes.
In terms of the underlying technology and the manufacturers behind them, the AI gold rush has attracted interest from every corner of the technology world. This has ranged from GPU and CPU companies to FPGA firms, custom ASIC markers, and more. There is a need for inference at the edge, inference at the cloud, training in the cloud – AI processing at every level, served by a variety of processors. But among all of these facets of AI, the most lucrative market of all is the market at the top of this hierarchy: the datacenter. Expansive, expensive, and still growing by leaps and bounds, the datacenter market is the ultimate feast or famine setup, as operators are looking to buy nothing short of massive quantities of discrete processors. And now, one of the last juggernauts to sit on the sidelines of the datacenter AI market is finally making its move: Qualcomm
This morning at their first Qualcomm AI Day, the 800lb gorilla of the mobile world announced that they are getting into the AI accelerator market, and in an aggressive way. At their event, Qualcomm announced their first discrete dedicated AI processors, the Qualcomm Cloud AI 100 family. Designed from the ground up for the AI market and backed by what Qualcomm is promising to be an extensive software stack, the company is throwing their hat into the ring for 2020, looking to establish themselves as a major vendor of AI inference accelerators for a hungry market.
But before we too far into things here, it’s probably best to start with some context for today’s announcement. What Qualcomm is announcing today is almost more of a teaser than a proper reveal – and certainly far from a technology disclosure. The Cloud AI 100 family of accelerators are products that Qualcomm is putting together for the 2020 timeframe, with samples going out later this year. In short, we’re probably still a good year out from commercial products shipping, so Qualcomm is playing things cool, announcing their efforts and their rationale behind them, but not the underlying technology. For now it’s about making their intentions known well in advance, especially to the big customers they are going to try to woo. But still, today’s announcement is an important one, as Qualcomm has made it clear that they are going in a different direction than the two juggernauts they’ll be competing with: NVIDIA and Intel.
The Qualcomm Cloud AI 100 Architecture: Dedicated Inference ASIC
So what exactly is Qualcomm doing? In a nutshell, the company is developing a family of AI inference accelerators for the datacenter market. Though not quite a top-to-bottom initiative, these accelerators will come in a variety of form factors and TDPs to fit datacenter operator needs. And within this market Qualcomm expects to win by virtue of offering the most efficient inference accelerators on the market, offering performance well above current GPU and FPGA frontrunners.
The actual architectural details on the Cloud AI 100 family are slim, however Qualcomm has given us just enough to work with. To start with, these new parts will be manufactured on a 7nm process – presumably TSMC’s performance-oriented 7nm HPC process. The company will offer a variety of cards, but it’s not clear at this time if they are actually designing more than one processor. And, we’re told, this is an entirely new design built from the ground up; so it’s not say a Snapdragon 855 with all of the AI bits scaled up.
In fact it’s this last point that’s probably the most important. While Qualcomm isn’t offering architectural details for the accelerator today, the company is making it very clear that this is an AI inference accelerator and nothing more. It’s not being called an AI training accelerator, it’s not being called a GPU, etc. It’s only being pitched for AI inference – efficiently executing pre-trained neural networks.
This is an important distinction because, while the devil is in the details, Qualcomm’s announcement very strongly points to the underlying architecture being an AI inference ASIC – ala something like Google’s TPU family – rather than being a more flexible processor. Qualcomm is of course far from the first vendor to build an ASIC specifically for AI processing, but while other AI ASICs have either been focused at the low-end of the market or reserved for internal use (Google’s TPUs again being the prime example), Qualcomm is talking about an AI accelerator to be sold to customers for datacenter use. And, relative to the competition, what they are talking about is much more ASIC-like than the GPU-like designs everyone is expecting in 2020 out of front-runner NVIDIA and aggressive newcomer Intel.
That Qualcomm’s Cloud AI 100 processor design is so narrowly focused on AI inference is critical to its performance potential. In the processor design spectrum, architects balance flexibility with efficiency; the closer to a fixed-function ASIC a chip is, the more efficient it can be. Just as how GPUs offered a massive leap in AI performance over CPUs, Qualcomm wants to do the same thing over GPUs.
The catch, of course, is that a more fixed-function AI ASIC is giving up flexibility. Whether that’s the ability to handle new frameworks, new processing flows, or entirely new neural networking models remains to be seen. But Qualcomm will be making some significant tradeoffs here, and the big question is going to be whether these are the right tradeoffs, and whether the market as a whole is ready for a datacenter-scale AI ASIC.
Meanwhile, the other technical issue that Qualcomm will have to tackle with the Cloud AI 100 series is the fact that this is their first dedicated AI processor. Admittedly, everyone has to start somewhere, and in Qualcomm’s case they are looking to translate their expertise in AI at the edge with SoCs into AI at the datacenter. The company’s flagship Snapdragon SoCs have become a force to be reckoned with, and Qualcomm thinks that their experience in efficient designs and signal processing in general will give the company a significant leg up here.
It doesn’t hurt either that with the company’s sheer size, they have the ability to ramp up production very quickly. And while this doesn’t help them against the likes of NVIDIA and Intel – both of which can scale up at TSMC and their internal fabs respectively – it gives Qualcomm a definite advantage over the myriad of smaller Silicon Valley startups that are also pursuing AI ASICs.
Why Chase the Datacenter Inferencing Market?
Technical considerations aside, the other important factor in today’s announcement is why Qualcomm is going after the AI inference accelerator market. And the answer, in short, is money.
Projections for the eventual size of the AI inferencing market vary widely, but Qualcomm buys in to the idea that datacenter inference accelerators alone could be a $17 billion market by 2025. And if this proves to be true, then it would represent a sizable market that Qualcomm would otherwise be missing out on. One that would rival the entirely of their current chipmaking business.
It’s also worth noting here that this is explicitly the inference market, and not the overall datacenter inference + training market. This is an important distinction because while training is important as well, the computational requirements for training are very difference from inferencing. While accurate inferencing can be performed with relatively low-precision datatypes like INT8 (and sometimes lower), currently most training requires FP16 or more. Which requires a very different type of chip, especially when we’re talking about ASICs instead of something a bit more general purpose like a GPU.
This also leans into scale: while training a neural network can take a lot of resources, it only needs to be done once. Then it can be replicated out many times over to farms of inference accelerators. So as important as training is, potential customers will simply need many more inference accelerators than they will training-capable processors.
Meanwhile, though not explicitly said by the company, it’s clear that Qualcomm is looking to take down market leader NVIDIA, who has built a small empire out of AI processors even in these early days. Currently, NVIDIA’s Tesla T4, P4, and P40 accelerators make up the backbone of datacenter AI inference processors, with datacenter revenues as a whole proving to be quite profitable for NVIDIA. So even if the total datacenter market doesn’t grow quite as projected, it would still be quite lucrative.
Qualcomm also has to keep in mind the threat from Intel, who has very publicly telegraphed their own plans for the AI market. The company has several different AI initiatives, ranging from low-power Movidius accelerators to their latest Cascade Lake Xeon Scalable CPUs. However for the specific market Qualcomm is chasing, the biggest threat is probably Intel’s forthcoming Xe GPUs, which are coming out of the company’s recently rebuilt GPU division. Like Qualcomm, Intel is gunning for NVIDIA here, so there is a race for the AI inference market that none of the titans wish to lose.
Making It to the Finish Line
Qualcomm’s ambitions aside, for the next 12 months or so, the company’s focus is going to be on lining up its first customers. And to do this, the company has to show that it’s serious about what it’s doing with the Cloud AI 100 family, that it can deliver on the hardware, and that it can match the ease of use of rivals’ software ecosystems. None of this will be easy, which is why Qualcomm has needed to start now, so far ahead of when commercial shipments begin.
While Qualcomm has had various dreams of servers and the datacenter market for many years now, perhaps the most polite way to describe those efforts are “overambitious.” Case in point would be Qualcomm’s Centriq family of ARM-based server CPUs, which the company launched with great fanfare back in 2017, only for the entire project to collapse within a year. The merits of Centriq aside, Qualcomm is still a company that is largely locked to mobile processors and modems on the chipmaking side. So to get datacenter operators to invest in the Cloud AI family, Qualcomm not only needs a great plan for the first generation, but a plan for the next couple of generations beyond that.
The upshot here is that in the young, growing market for inference accelerators, datacenter operators are more willing to experiment with new processors than they are, say, CPUs. So there’s no reason to believe that the Cloud AI 100 series can’t be at least moderately successful right off the bat. But it will be up to Qualcomm to convince the otherwise still-cautious datacenter operators that Qualcomm’s wares are worth investing so many resources into.
Parallel to this is the software side of the equation. A big part of NVIDIA’s success thus far has been in their AI software ecosystem – itself is an expansion of their decade-old CUDA ecosystem – which has vexed GPU rival AMD for a while now. The good news for Qualcomm is that the most popular frameworks, runtimes, and tools have already been established; TensorFlow, Caffe2, and ONNX are the big targets, and Qualcomm knows it. Which is why Qualcomm is promising an extensive software stack right off the bat, because nothing less than that will do. But Qualcomm does have to get up to speed very quickly here, as how well their software stack actually works can make or break the whole project. Qualcomm needs to deliver good hardware and good software to succeed here.
But for the moment at least, Qualcomm's announcement today is a teaser – a proclamation of what’s to come. The company has developed a very ambitious plan to break into the growing AI inference accelerator market, and to deliver a processor significantly unlike anything else on the open market. And while getting from here to there is going to be a challenge, as one of the titans of the processor world Qualcomm is among the most capable out there, both in funding and engineering resources. So it’s as much a question of how badly Qualcomm wants the inference accelerator market as it is their ability to develop processors for it; and how well they can avoid the kind of missteps that have sunk their previous server processor plans.
Above all else, however, Qualcomm won’t simply take the inference accelerator market: they’re going to have to fight for it. This is NVIDIA’s market to lose and Intel has eyes on it as well, never mind all the smaller players from GPU vendors, FPGA vendors, and other ASIC players. Any and all of which can quickly rise and fall in what’s still a young market for an emerging technology. So while it’s still almost a year off, 2020 is quickly shaping up to be the first big battle for the AI accelerator market.
26 Comments
View All Comments
p1esk - Tuesday, April 9, 2019 - link
This should be called "a paper launch of a paper launch". To provide a perspective, Intel announced Nervana NNP-I accelerator back in 2017, saying "first silicon will be shipped by the end of the year". Still not out.p1esk - Tuesday, April 9, 2019 - link
https://www.anandtech.com/show/11942/intel-shippin...The Hardcard - Tuesday, April 9, 2019 - link
I imagine one of the challenges is that this is a really fast moving target. In 2017 this was being done in FP32 and shifting into 16 bits. But chips for that are already obsolete. Training in 8 bits, inference in 4 bits. Releasing a new silicon family that can't do that might be a waste of investment, even if you have already finalized.The good thing is, it sounds like those are going to be the limits at least for a while.
p1esk - Tuesday, April 9, 2019 - link
The problem is you can't just build a chip that only supports 8 bit for training and 4 bit for inference. There will always be models/tasks which either need higher precision, or work well with lower precision. DL hardware must remain flexible (with support for at least 16 bits). Note that latest Nvidia chips support precision all the way to INT1, with linear scaling in performance.The Hardcard - Wednesday, April 10, 2019 - link
Oh, I agree. I would expect that 32-bit math to still be there. My point is that the difficulty in selling products that can’t do 4-bit calculations might explain why some previously announced products might be delayed for release.There are probably other factors - instruction set, memory models, and communication protocols - that also need to try to keep up with this quickly advancing field.
p1esk - Wednesday, April 10, 2019 - link
Also, developing an inference-only chip for the market 2-4 years from now is risky, because as you said, ML field is a fast moving target. What if online learning becomes popular/effective (models that continuously learns from real-time data, e.g. [1])? And maybe you need 16 bit precision to make it work (maybe not, but what if), and these chips just don't support it.p1esk - Wednesday, April 10, 2019 - link
https://arxiv.org/abs/1903.08671rahvin - Tuesday, April 9, 2019 - link
Intel at least has AI silicon already available from the their purchase. This is Qualacomm's first dedicated silicon and it's occuring after the activist investor got them to drop the server chip because it cost too much to develop. Unless they show real silicon I'd take any promises of future silicon with a truckload of salt.GreenReaper - Tuesday, April 9, 2019 - link
It's perhaps more accurate to say that Qualcomm wants *everyone else* to buy into the idea of datacenter inference accelerators. Something tells me revenue won't quite meet up with projections!beginner99 - Wednesday, April 10, 2019 - link
Fully agree. Since inference doesn't take much calculation power at all I even fail to see the need for these. Ar ethere apps that run models in the cloud? then why do we get AI hardware on phones? And stuff like mobilenet?